Machine Learning and the evolution algorithmic
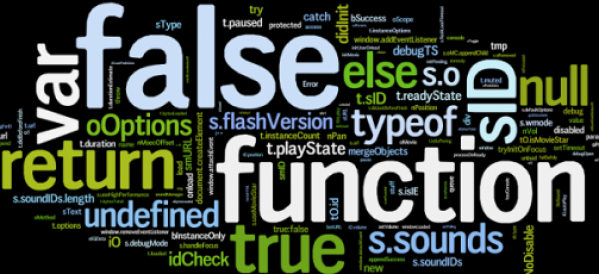
Analytical applications, in general, were directed to the academic segment, currently assist us in everyday situations
The world is constantly evolving. But it is clear that many of the technological solutions used today are advancing technologies that our parents and grandparents used in the past. Analytical applications, in general, were directed to the academic segment, currently assist us in everyday situations. For example, when requesting a financial loan we do not realize that an analytical technology is used to authorize or not the credit to an individual. Personal information is compared with the thousands of other clients who have gone through the same situation. Overall, the data and habits of that individual are similar to those of people who honored their commitments, it will be released credit.
But how to identify this similarity among thousands of people and individual members? How to avoid the most to do injustice to deny credit to customers who would honor the commitment to pay on time or releasing the requested amount to a person who does not honor? The answer to these questions is found in the ‘Analytical Models’. There are several strategies to create these models and a widely used is the Machine Learning.
What is Machine Learning?
Previously, this type of methodology was something distant and more like science fiction, but today is fundamental for business. Machine Learning is an area of computer science, created from research related to Artificial Intelligence. The increasing use of these methods is fully related to the strong computational growth. If in the past people tried to solve complex formulas almost manually, today employ the technology to automate the construction of analytical models that use algorithms to learn from data interactively. In other words, the idea is that these algorithms are almost self-sufficient with the least possible human intervention.
What differs Machine Learning of Statistics or Statistical Models?
Machine Learning and Statistics are equivalent in some respects. This means that the results obtained with a method can be compared to each other. However, when it comes to create Statistical Models the goal is to learn something related to the data, that is, gain insights from existing information. Already Machine Learning, rather than simply trying to understand what occurs in the data, what we have is the ‘creation of examples’ or ‘rules’, and each execution of the algorithm, it is able to learn and improve from examples. The interactive aspect of Machine Learning is important because the models are improved by learning from internal calculations producing better results.
Machine Learning of importance nowadays
The Machine Learning has historical importance. There are reports that this set of techniques was born during World War II and has been refined to the present day. To give you an idea, this methodology was already present, for example, SAS since the 80s this time, using techniques from Machine Learning, already sought to find behavioral profiles in order to identify fraudsters.
The extraordinary surge in the use of this methodology is given for a few reasons. First, because the amount of data is increasing at an unprecedented rate. For most organizations, the challenge is to extract valuable information from huge data volumes from a huge variety of different sources. Another point is the storage options of this information – now more affordable or even free. Furthermore, the computational processing power has never been cheaper or more powerful.
This means that, with the right data, the right technologies, and appropriate analysis, it is possible to produce models that can quickly analyze a large amount of data, regardless of its complexity and deliver results in less time, more accurate and with minimal human intervention. The result? Forecasts of high value that can guide the best decisions.
Applications for Machine Learning are numerous, such as: detection of fraud; online offers recommendations; advertisements in real time on the web and mobile; sentiment analysis based on textual sources of social networks; credit scoring; failure prediction in equipment; new pricing models; intrusion detection in networks; Analysis writing patterns; among others.
We can say that the central idea is to use a high-performance technology with interactive aspect. What makes the use of Machine Learning advantageous is the ability to create systems that learn from the data itself getting the best results with minimal human intervention, generating highly effective results and enabling strategic decisions, including, in real time.
Full article: http://cio.com.br/